Using drones to enable AI in space
![Drone controlled by a Guidance & Control Network inside the TU Delft Cyberzoo [Credits to Robin Ferede and Christophe De Wagter (Micro Air Vehicle Laboratory)]. We examine drone racing as an optimal gym to test such neural architecures on real robotic platforms, aiming to boost confidence in their application for future space-base missions.](/gsp/ACT/images/news/drone_mavlab.png)
The latest issue of the Science Robotics journal features our work on 'Optimality Principles in Spacecraft Neural Guidance and Control' The research is also featured in ESA's news as Drone racing prepares neural-network AI for space and proposes that future space missions could utilize entirely neural-based on-board optimal guidance and control systems.
We emphasize the advancements in training end-to-end neural architectures for applications such as interplanetary mission guidance, planetary landings, and close-proximity operations. These sophisticated neural models are capable of learning optimal strategies to significantly enhance the autonomy and efficiency of spacecraft and unmanned aerial vehicles. This is important since spacecraft and drones designed for solar system exploration must efficiently utilize onboard resources, therefore sensorimotor actions are often derived from high-level, quantifiable optimality principles using optimal control theory.
By implementing Guidance & Control Networks (G&CNets), optimal actions can be computed in real-time, thereby increasing mission robustness. Additionally, drone racing is examined as an ideal test environment for these architectures, sharing similarities with spacecraft missions in limited onboard computational capabilities and control structures, despite differing in dynamical timescales and uncertainties. We showcase a drone which uses a G&CNET in the top Figure. The bottom Figure highlights how one of the problems associated with machine learning, namely the time-consuming generation of large training datasets, is addressed.
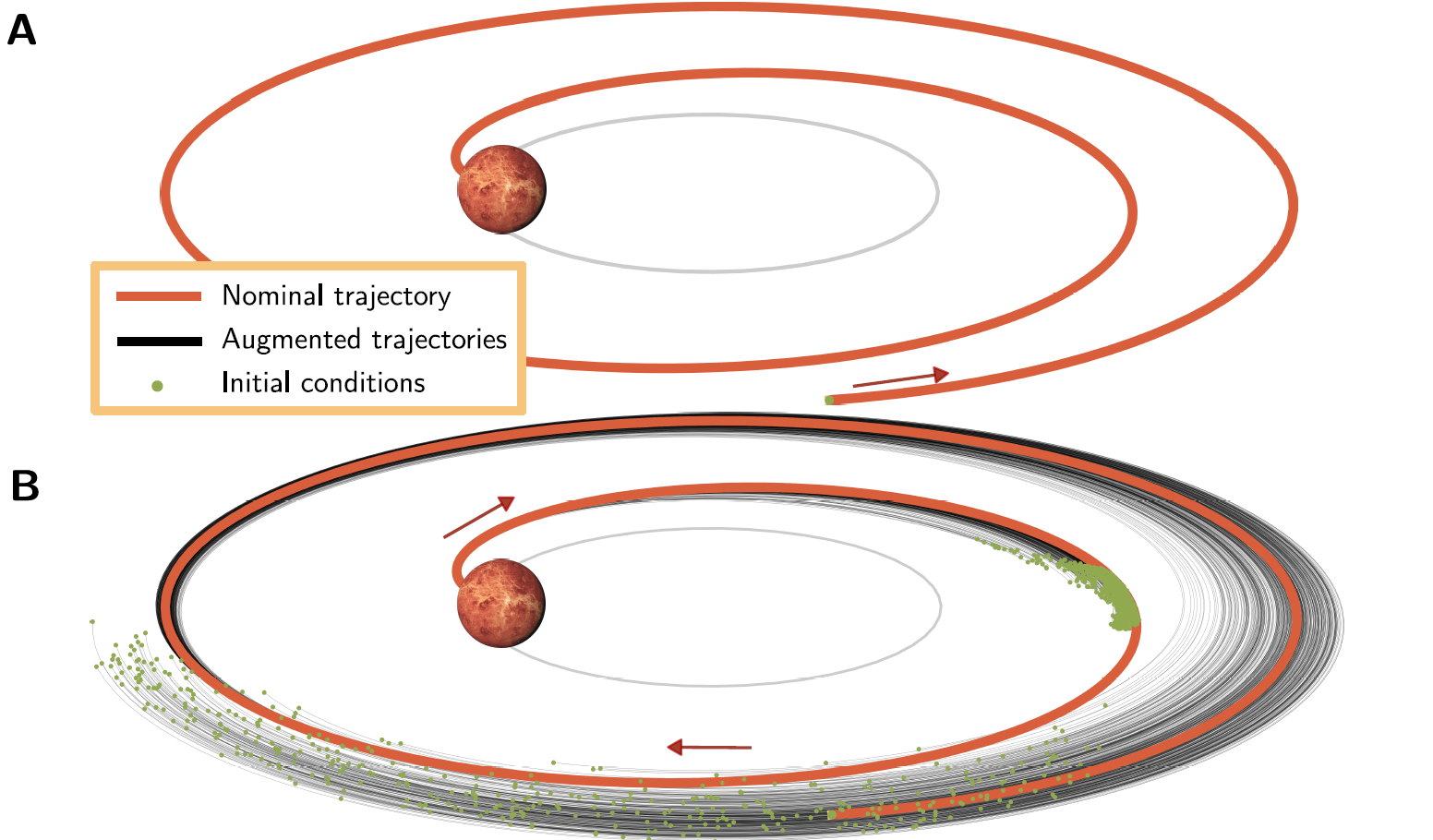